By Julia Schwarz
All intelligent organisms have a nervous system, a way for communication to flow between the brain and the motor system and vice versa. Researchers at Princeton have taken a first step in developing this type of coordination for mechanical AI systems using the tools of machine learning.
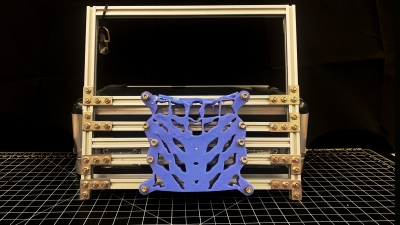
The research is a collaboration between graduate student Deniz Oktay and Ryan P. Adams, professor of computer science, along with postdoctoral researchers Mehran Mirramezani and Eder Medina. Oktay presented the work as a poster at the International Conference on Learning Representations.
“Part of what's amazing about biological systems is that bodies and brains co-evolved over billions of years and work together incredibly efficiently,” said Adams. This coordinated evolution has resulted in systems that can execute novel tasks with very little effort, or even involuntarily. Think of a baby learning to grasp a finger long before she can talk.
The researchers have tried to approximate this co-evolution by developing a neural network alongside a physical structure. The structure in this case is a molded piece of rubber with a patchwork of carefully designed honeycomb-like cutouts, what the researchers call mechanical metamaterials. Those cutouts have a unique and complicated geometry, giving the neural network a challenging physical structure to learn.
After training the neural network, Oktay assigned it a series of tasks, each testing its ability to manipulate this complex material. Because the neural network and the material are designed for each other, the network can learn to control the structure without detailed instructions. “They can act in tandem,” said Oktay.
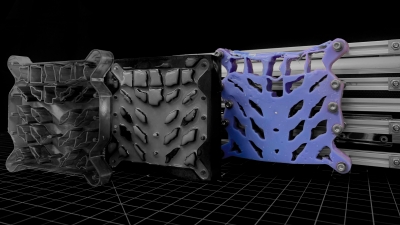
This is a significant departure from the current approach to AI and robotics. “The common approach right now is to build a very well-engineered robot arm and layer a brain on top of it,” said Adams. Because the robotic arm and the AI brain are not designed for each other, the result is a system that requires a huge amount of sophisticated mechanical engineering and computing power to perform even the simplest tasks.
The new research could be a first step to creating a more efficient system. In principle, the technique could work with any kind of flexible material, even fluids. For now, the neural network controls the material in a virtual simulation, and the researchers verify the solutions manually using the rubber device. They plan to test three dimensional forms and more complex structures next. “We'd love to eventually, one day, build things that can walk and swim and fly,” said Adams.
The research, “Neuromechanical Autoencoders: Learning to Couple Elastic and Neural Network Nonlinearity” was presented as a poster at the International Conference on Learning Representations, May 1-5, 2023. The work was supported by the National Science Foundation, the Computing Research Association, and a Siemens Ph.D. fellowship.