By Julia Schwarz
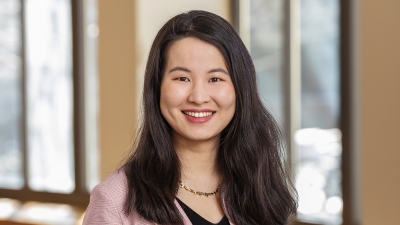
Lydia Liu, an expert on the social impacts of machine learning, joined the computer science faculty as an assistant professor in January 2024.
Liu’s research focuses on algorithmic decision-making and data-driven predictions, with an emphasis on how these tools affect individuals. Predictive algorithms are used across critical sectors including health care, education and government to show whether, for example, a student is likely to graduate or whether a defendant is likely to reoffend.
It’s a very human impulse to want to predict the future, said Liu. “We’ve been doing it since the Oracle at Delphi in Ancient Greece.” she said. “And now we’re using mathematical algorithms to make predictions about individual people."
After decades of study, researchers now know a lot about how to evaluate the accuracy and robustness of an algorithm and the fairness of the predictions, said Liu. Much of her work focuses on the next step: how people and organizations use these predictions. “There's so much focus on having accurate predictions that what to do with that information sometimes becomes an afterthought,” she said.
Although her research often explores the mathematical foundations of these questions, she is passionate about applying these insights to the domain of education. While doing doctoral work in computer science at the University of California-Berkeley, Liu collaborated with education researchers to look at whether machine learning tools could help educators and staff optimize admissions, lower student dropout rates, and facilitate massive open online courses. Currently, she’s evaluating the impact of data-driven advising on student success outcomes.
Her research has shown that the quality of the prediction tool is as important as the amount of human effort put into using those predictions to improve outcomes. Where students become more successful, she said, it’s never just due to the robustness of the algorithm. It’s the result of significant human effort put into evaluating the data and then deciding how to use it.
Liu did her undergraduate work at Princeton and majored in operations research & financial engineering. She has always been interested in the social applications of engineering, she said. In graduate school she initially did purely mathematical work on algorithm design but kept circling back to the question of what machine learning was for. “I kept asking myself: If we make the algorithm better,” she said, “who is that going to help?”
While computer science researchers often focus on building tools, Liu is interested in evaluating the broader impacts of such tools once they’re built, an approach that is gaining momentum across the discipline. “We want to think about what's at stake,” she said.
In Fall 2024, Liu will be teaching a graduate-level course on AI, Society, and Education, which will look broadly at algorithmic decision-making and ask students to think creatively about how to evaluate AI systems. And in Spring 2025, she will be teaching COS 324: Introduction to Machine Learning.
As an associated faculty member at the Center for Information Technology Policy, she sees Princeton as an ideal place to continue her research into both the scientific and normative foundations of data-driven predictions. “Princeton makes a lot of great interdisciplinary conversations possible,” she said.