PNI/CS Special Seminar
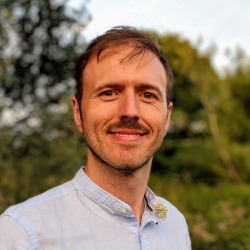
The brain is an unparalleled learning machine, yet the principles that govern learning in the brain remain unclear. In this talk I will suggest that depth–the serial propagation of signals–may be a key principle sculpting learning dynamics in the brain and mind. To understand several consequences of depth, I will present mathematical analyses of the nonlinear dynamics of learning in a variety of simple solvable deep network models. Building from this theoretical work, I will trace implications for the development of human semantic cognition, showing that deep but not shallow networks exhibit hierarchical differentiation of concepts through rapid developmental transitions and ubiquitous semantic illusions between such transitions. Finally, turning to rodent systems neuroscience, I will show that deep network dynamics can account for individually variable yet systematic transitions in strategy as mice learn a visual detection task over several weeks. Together, these results provide analytic insight into how the statistics of an environment can interact with nonlinear deep learning dynamics to structure evolving neural representations and behavior over learning.
Bio: Andrew Saxe is a Professorial Research Fellow at the Gatsby Computational Neuroscience Unit and Sainsbury Wellcome Centre at UCL. He was previously an Associate Professor in the Department of Experimental Psychology at the University of Oxford. His research focuses on the theory of deep learning and its applications to phenomena in neuroscience and psychology. His work has been recognized by the Robert J. Glushko Dissertation Prize from the Cognitive Science Society, the Blavatnik UK Finalist Award in Life Sciences, and a Schmidt Science Polymath award. He is also a CIFAR Azrieli Global Scholar in the CIFAR Learning in Machines & Brains programme.
To request accommodations for a disability please contact Yi Liu, irene.yi.liu@princeton.edu, at least one week prior to the event.